Effect size is a measure that shows how strong a relationship is between variables or how large the difference is…
Dissertation data analysis involves examining, interpreting, and presenting data to answer your research questions or test hypotheses. It transforms raw data into meaningful insights, forming the backbone of your research findings. This section is crucial because it demonstrates your ability to critically analyze data and connect it to your study’s objectives, contributing to the overall credibility of your dissertation.
A well-structured data analysis ensures clarity, logical flow, and alignment with your research methodology, making it easier for readers to understand your findings. Proper organization also helps avoid misinterpretation and emphasizes the significance of your results.
This blog article focuses on providing 10 actionable tips to help you craft an effective data analysis chapter for your dissertation, thesis, or research project. Whether you’re analyzing quantitative or qualitative data, these tips will guide you in structuring, interpreting, and presenting your results with precision and clarity.
What is Dissertation Data Analysis?
Dissertation data analysis is the process of examining and interpreting data collected during research to uncover patterns, relationships, or trends that address the study’s objectives or test its hypotheses. It involves transforming raw data into meaningful insights, providing the foundation for your research conclusions and recommendations.
Data analysis plays a critical role in answering research questions by linking theoretical concepts to empirical evidence. It ensures that your findings are grounded in evidence, enhancing the validity and reliability of your dissertation.
The approach to data analysis depends on your research methodology. Quantitative data analysis focuses on numerical data, using statistical techniques such as regression, ANOVA, or correlation to identify relationships. In contrast, qualitative data analysis explores non-numerical data, employing methods like thematic analysis or coding to uncover themes, narratives, or meanings. Understanding these differences ensures you select the right analytical techniques for your research.
10 Tips for Writing a Stellar Dissertation Data Analysis Chapter
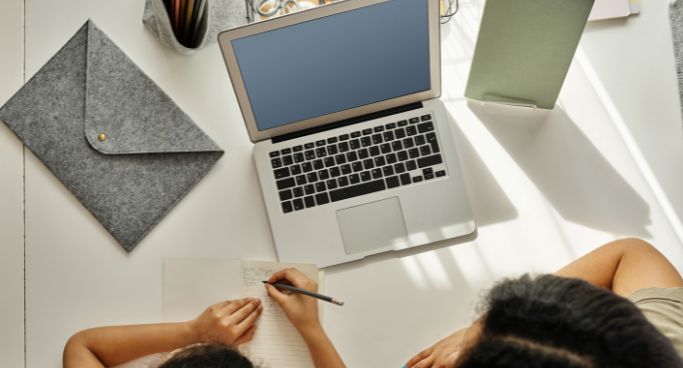
Writing the data analysis chapter of your dissertation can feel overwhelming, but it’s also one of the most rewarding parts of your research journey. This is where you uncover the insights hidden within your data and bring your research to life. To ensure your analysis is impactful and professionally presented, follow these ten actionable tips, each designed to guide you toward a stellar dissertation.
Tip 1: Clearly Define Your Research Questions or Hypotheses
Your research questions or hypotheses serve as the compass for your entire data analysis process. They provide direction, ensuring that your analysis remains focused and aligned with your study’s objectives. Before diving into the analysis, revisit your research questions to ensure they’re specific, measurable, and relevant. For example, instead of asking a broad question like, “What affects employee performance?” narrow it to something like, “How does remote work influence employee productivity in the healthcare sector?”
Clearly defined questions also guide how you interpret your data. They help you determine which variables to analyze, what statistical tests to apply, and how to structure your results. Without a clear framework, you risk producing findings that lack coherence or relevance. By aligning your analysis with your research questions, you’ll make your data interpretation stronger and more meaningful to your dissertation’s objectives.
Tip 2: Choose the Right Analytical Tools and Software
Selecting the right tools for your data analysis is critical to achieving accurate and reliable results. With so many software options available, it’s essential to choose one that suits your data type and research methodology. For quantitative data, popular tools like SPSS, R, and STATA are excellent for statistical analyses, offering features such as regression, ANOVA, and descriptive statistics. If you’re working with qualitative data, consider NVivo or Atlas.ti for coding and thematic analysis.
When selecting tools, consider your familiarity with the software and the complexity of your data. If you’re a beginner, a user-friendly option like SPSS might be best, whereas more advanced users might prefer R for its flexibility and depth. Don’t forget to leverage online tutorials and resources to familiarize yourself with your chosen tool. The right software can save time and help you present your findings more effectively.
Tip 3: Organize and Clean Your Data Thoroughly
Data cleaning might not be the most glamorous part of research, but it’s one of the most crucial. Raw data often contains errors, inconsistencies, or missing values that can compromise the validity of your analysis. Start by organizing your dataset into a structured format—rows for observations and columns for variables—to make it easier to manage.
Cleaning involves checking for and addressing issues such as duplicate entries, outliers, and incomplete responses. For quantitative data, ensure numerical variables are formatted correctly and free of errors. For qualitative data, review transcripts for accuracy and clarity. Tools like Excel or Python can be used for basic cleaning tasks, while specialized software like SPSS or R is ideal for more advanced preprocessing. A well-organized dataset ensures that your analysis is smooth and your results are reliable.
Tip 4: Use Appropriate Analytical Methods
Choosing the correct analytical methods is critical for ensuring your results align with your research objectives. Quantitative studies often involve statistical techniques such as t-tests, regression analysis, or ANOVA to identify relationships or differences between variables. On the other hand, qualitative research focuses on methods like thematic analysis, coding, or content analysis to uncover patterns and themes in textual data.
The key is to match your methods to your research design. For instance, if your study examines the impact of an intervention, you might use pre- and post-test comparisons. For exploratory qualitative research, thematic analysis might be more suitable. Carefully justify your chosen methods in your dissertation, showing how they align with your data and objectives. This not only strengthens your analysis but also demonstrates your methodological rigor to your readers.
Tip 5: Structure Your Data Analysis Chapter Clearly
A well-structured dissertation data analysis chapter is essential for communicating your findings effectively. Divide your analysis into logical sub-sections, such as descriptive statistics, inferential statistics, and interpretations. Begin with an overview of your dataset, providing details like sample size and demographic characteristics.
Next, present your findings in a systematic manner, focusing on one research question or hypothesis at a time. Use subheadings to improve readability and ensure your results follow a clear and logical flow. For example, start with descriptive statistics to provide context, then move on to inferential analyses to address your research questions. A clear structure helps readers navigate your analysis and strengthens the overall impact of your work.
Tip 6: Visualize Your Data Effectively
Data visualization is a powerful tool for making your findings more accessible and engaging. Charts, graphs, and tables can highlight key patterns and trends, making it easier for readers to understand complex data. For instance, use bar charts to compare categorical data or scatter plots to show relationships between variables.
Tools like Excel, Tableau, or Python can help you create professional and visually appealing graphics. When designing visualizations, prioritize clarity and simplicity—avoid overcrowding graphs with too much information. Always include labels, legends, and captions to provide context. By effectively visualizing your data, you can communicate your findings more persuasively and leave a lasting impression on your readers.
Tip 7: Interpret Results with Precision
Interpreting your results accurately is as important as conducting the analysis itself. Avoid simply describing your findings; instead, explain what they mean in the context of your research questions. For example, if a regression analysis shows a significant positive relationship between variables, discuss its implications and potential causes.
Be cautious not to overgeneralize or make unsupported claims. Stick to what the data reveals and avoid inferring beyond the scope of your study. Highlight anomalies or unexpected findings and provide possible explanations for them. Precise interpretation not only strengthens your argument but also demonstrates your critical thinking skills and deep understanding of your research.
Tip 8: Compare Findings with Existing Literature
Your analysis doesn’t exist in isolation; it’s part of a broader academic conversation. Contextualize your results by comparing them with findings from previous studies. This helps highlight the significance of your research and shows how it contributes to existing knowledge.
Identify whether your findings support or contradict prior research, and discuss possible reasons for any discrepancies. For instance, differences in sample size, context, or methodology could explain varying results. By situating your analysis within the existing literature, you demonstrate your research’s relevance and enhance its academic value.
Tip 9: Keep Your Writing Clear and Concise
Clear and concise writing is essential for effectively communicating your data analysis. Avoid using jargon or overly complex language that might confuse your readers. Instead, explain your findings in simple, straightforward terms, focusing on what the results mean rather than how they were obtained.
Use short paragraphs and bullet points to break down complex information. Ensure your explanations are logical and free of redundancy. Remember, the goal is to make your analysis accessible to a diverse audience, including those unfamiliar with your specific field. Clear writing enhances your analysis’s readability and impact.
Tip 10: Proofread and Seek Expert Feedback
Before finalizing your data analysis section, take the time to proofread it thoroughly. Check for errors in calculations, inconsistencies in data presentation, and unclear explanations. Tools like Grammarly or Hemingway can help improve your writing quality.
Seeking feedback from your supervisor, peers, or professional dissertation experts can also provide valuable insights. They can help identify gaps in your analysis or suggest ways to improve clarity and coherence. Remember, a polished and error-free data analysis section not only strengthens your dissertation but also leaves a positive impression on your readers.
Common Mistakes to Avoid in Dissertation Data Analysis
When writing your dissertation data analysis, avoiding common pitfalls is as crucial as implementing best practices. Misinterpreting statistical results ranks among the most frequent mistakes. For example, drawing conclusions that are not supported by your statistical tests can undermine the validity of your research. It’s essential to accurately interpret p-values, confidence intervals, and other metrics in alignment with your research objectives.
Another prevalent mistake is overloading the section with unnecessary details. While it’s tempting to include every single piece of analysis, doing so can overwhelm readers and obscure your key findings. Instead, focus on presenting insights that directly address your research questions and hypotheses.
Lastly, ignoring anomalies in your data can lead to biased or incomplete conclusions. Outliers or unexpected patterns may hold valuable insights or indicate issues with data collection or entry. Always document and analyze anomalies, ensuring transparency in your research process.
To further refine your dissertation data analysis, explore our comprehensive guide on the top 10 mistakes to avoid in dissertation data analysis. This resource provides detailed advice to help you navigate potential pitfalls and enhance the quality of your analysis.
Conclusion
A strong dissertation data analysis chapter is the cornerstone of a successful dissertation. It not only demonstrates your ability to interpret data but also directly addresses your research questions, making it a critical component of your academic work. By following the tips shared in this guide—from defining clear research questions to proofreading and seeking expert feedback—you can craft a data analysis chapter that is both impactful and insightful.
Remember, the goal is to present your findings clearly and concisely while ensuring they are well-supported by evidence. Taking the time to organize, clean, and analyze your data thoroughly will enhance the credibility of your research. Additionally, visualizing your data effectively and interpreting results with precision will help you communicate your findings in a compelling way.
Need Help with Your Dissertation Data Analysis?
Don’t let the challenges of dissertation data analysis hold you back! Whether you’re struggling with complex statistical methods, software tools, or structuring your analysis, our Dissertation Data Analysis Help is here to help. We specialize in providing tailored assistance to ensure your data analysis is accurate, insightful, and aligned with your research objectives.
Take the stress out of your dissertation journey by partnering with professionals who understand the intricacies of data analysis. Contact us today to learn how we can assist you in achieving your academic goals. Click below to get started!