Effect size is a measure that shows how strong a relationship is between variables or how large the difference is…
A data analysis plan is a critical component of any dissertation. Specifically, it serves as a blueprint for analyzing and interpreting research findings. A data analysis plan also ensures your analysis aligns with the study’s objectives, research questions, and hypotheses. This provides a clear roadmap for making sense of the data. Thus, a well-structured plan not only enhances the credibility of your research but also streamlines the analysis process, saving time and reducing errors.
In this article, we’ll guide you through the essentials of writing an effective dissertation data analysis plan. You’ll learn how to outline your analysis objectives, choose appropriate methods, and address common challenges. By the end, you’ll have the tools to craft a comprehensive plan that sets your research up for success. Whether you’re working with qualitative, quantitative, or mixed methods data, this guide will help you navigate the process with confidence.
What Is a Dissertation Data Analysis Plan?
A dissertation data analysis plan is a structured outline that details how you will process, analyze, and interpret your research data. It acts as a bridge between data collection and the interpretation of results. This ensures that the analysis is systematic, rigorous, and aligned with the study’s objectives. By providing a clear roadmap, the data analysis plan helps researchers identify the most appropriate methods and tools for analyzing their data.
The primary purpose of a data analysis plan is to ensure that the analysis directly addresses the research questions and hypotheses. This alignment is crucial for producing valid and meaningful results that contribute to answering the study’s central problem. A well-defined plan also anticipates potential challenges, such as missing data or outliers, and includes strategies to address them. Ultimately, a strong data analysis plan provides clarity, consistency, and focus, enabling researchers to draw credible and actionable conclusions from their findings.
Why is a Data Analysis Plan Important?
A data analysis plan is essential for maintaining methodological rigor in your dissertation. It outlines the exact steps you will take to analyze your data, ensuring that your methods align with your research objectives. This clarity helps you stay focused and consistent throughout the process. Without a plan, it’s easy to fall into common pitfalls like using inappropriate techniques or overlooking important details.
A clear plan also prevents inconsistent analysis. It keeps you organized, especially when working with large datasets or complex research designs. By detailing each step in advance, you minimize errors and ensure that your findings are reliable and valid. This consistency builds trust in your results and makes your research credible.
Moreover, having a data analysis plan saves time. It guides your workflow, reducing unnecessary guesswork or delays. You know what to do, when to do it, and which tools to use. This efficiency allows you to spend more time interpreting results and less time troubleshooting problems. Thus, a data analysis plan is a roadmap that ensures rigor, prevents mistakes, and streamlines the research process. It sets a strong foundation for producing meaningful insights and achieving your dissertation goals.
Key Elements of a Dissertation Data Analysis Plan
Crafting a strong data analysis plan starts with understanding its key components. Each element serves as a building block, ensuring your analysis is clear, consistent, and aligned with your research goals. By addressing these areas thoroughly, you can create a roadmap that leads to meaningful and credible findings.
a. Restating Research Questions and Objectives
Your data analysis plan should begin with a restatement of your research questions and objectives. These guide your analysis by defining what you aim to discover or test. Clear research questions ensure that your analysis stays focused and relevant. For example, if your study examines the relationship between job stress and productivity, your analysis should directly address that relationship. Clearly stating objectives also helps in selecting the right methods and tools, ensuring that the results contribute to answering the core problem.
b. Identifying the Type of Data Collected
The type of data you collect—qualitative, quantitative, or mixed-methods—shapes your analysis approach. Quantitative data involves numerical information, often requiring statistical techniques like regression or ANOVA. Qualitative data, on the other hand, focuses on non-numeric insights such as themes or patterns from interviews, often analyzed through coding or thematic methods. Identifying your data type ensures the methods you choose are appropriate and effective.
c. Choosing Appropriate Analysis Methods
Selecting the right analysis methods is critical for extracting meaningful insights. For quantitative data, statistical tests like t-tests, chi-square tests, or ANOVA are commonly used. These methods help identify trends, relationships, or group differences. For qualitative data, approaches like thematic analysis, narrative analysis, or grounded theory help uncover deeper meanings and patterns. Choosing methods that align with your research design strengthens the validity of your findings.
d. Defining Tools and Software
Data analysis tools simplify complex computations and enhance accuracy. For quantitative research, software like SPSS, R, or Excel is ideal for statistical calculations. Qualitative data may require tools like NVivo or ATLAS.ti for coding and thematic analysis. Clearly defining the tools in your plan ensures efficiency and consistency, allowing you to focus on interpretation rather than manual calculations.
e. Handling Missing Data and Outliers
Handling missing data and outliers is crucial for maintaining the integrity of your analysis. Ignoring these issues can lead to skewed results. Techniques like imputation (replacing missing values with estimates) or excluding problematic data points can help. For outliers, consider methods like winsorizing or using robust statistical models that minimize their impact. Addressing these issues in your plan ensures your analysis is credible and reliable.
Step-by-Step Guide to Writing a Dissertation Data Analysis Plan
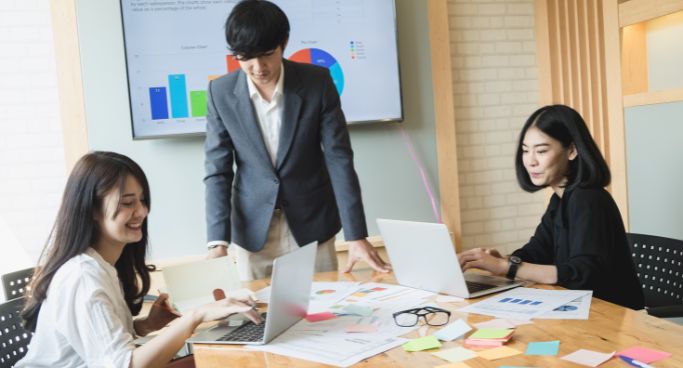
A strong data analysis plan is essential for turning raw data into meaningful insights. It acts as a roadmap that guides every step of your analysis process, ensuring clarity and consistency. By breaking it into manageable steps, you can approach the task with confidence and efficiency. Here’s a detailed guide to help you write a thorough and actionable data analysis plan.
Step 1: Outline Your Data Analysis Objectives
Start by clearly defining your analysis objectives. These objectives should align with your research questions and hypotheses, ensuring your analysis remains focused and relevant. For instance, if your research explores the impact of social media usage on academic performance, one objective might be to determine the correlation between screen time and GPA. Clearly outlining objectives helps you choose appropriate methods and avoid unnecessary detours. Include both primary and secondary objectives to capture the full scope of your analysis.
Step 2: Describe Your Data Collection Methods
Briefly summarize how you collected your data, as this directly informs your analysis approach. Mention whether you used surveys, interviews, experiments, or archival data, and highlight the rationale behind your choice. For example, if you conducted surveys to measure customer satisfaction, state how the questions were structured and what type of responses (quantitative or qualitative) you received. A clear description of your data collection methods ensures transparency and provides context for your analysis.
Step 3: Select the Appropriate Analytical Methods
Choose methods that match the type of data and the goals of your research. For quantitative data, common methods include t-tests for comparing groups, regression analysis for identifying relationships, or ANOVA for analyzing group differences. For qualitative data, consider coding, thematic analysis, or content analysis to uncover patterns and themes. Provide examples to illustrate your choices. For instance, if you’re studying interview responses, thematic analysis might help identify recurring themes. Clearly justify your methods to demonstrate their relevance to your study.
Step 4: Plan for Data Preparation
Data preparation is a crucial step that ensures the accuracy and reliability of your analysis. This involves cleaning the data by addressing missing values, correcting errors, and identifying outliers. For qualitative data, plan for coding and organizing the information into categories. For quantitative data, discuss steps like normalizing variables or creating composite scores. Detail how you will organize your dataset to facilitate smooth analysis. For instance, you might use software like SPSS or Excel to clean and sort your data before applying statistical tests.
Step 5: Create a Timeline for Analysis
A timeline ensures that your analysis stays on track and aligns with your dissertation deadlines. Divide your analysis into phases, such as data preparation, preliminary analysis, main analysis, and interpretation. Estimate how long each phase will take and include buffer time for unexpected challenges. For example, data cleaning might take a week, while running statistical tests could require two weeks. A well-thought-out timeline keeps your analysis process organized and manageable.
By following these steps, you can craft a comprehensive data analysis plan that lays the groundwork for credible and impactful research findings.
Common Challenges in Creating a Data Analysis Plan and How to Overcome Them
Creating a data analysis plan can be challenging, especially when faced with complex data and competing priorities. However, with preparation and the right strategies, you can overcome these obstacles and build a strong foundation for your research.
Difficulty Choosing Appropriate Methods
Selecting the right analysis methods can be overwhelming, particularly for first-time researchers. The wide range of techniques available for quantitative and qualitative data often adds to the confusion. To address this, seek guidance from your academic advisor or consult prior studies in your field. Look for examples that align with your research objectives and mimic their methodologies where applicable. Online resources, such as statistical guides or tutorials, can also help clarify when and how to use specific methods.
Managing Large Datasets
Working with large datasets can be daunting, especially when organizing, cleaning, and analyzing the data manually. To overcome this challenge, leverage analytical tools like SPSS, R, or Python, which can automate repetitive tasks and handle large volumes of data efficiently. Break the dataset into smaller, manageable subsets during the cleaning process to avoid feeling overwhelmed.
Addressing Ethical Considerations
Ensuring data privacy and ethical compliance is a critical part of any analysis plan. Challenges may arise when working with sensitive or personal data. Always adhere to institutional ethical guidelines and secure approval from relevant review boards. Use anonymization techniques to protect participants’ identities and ensure all data storage and sharing methods comply with privacy standards.
Tips for Writing an Effective Data Analysis Plan
Crafting a data analysis plan can feel overwhelming, but with the right approach, it becomes manageable and even empowering. A strong plan doesn’t just outline your steps—it communicates them clearly, ensuring your work is easy to follow and aligns with your research goals. Here are some practical tips to help you write an effective data analysis plan.
Use Clear and Concise Language
Clarity is crucial when writing a data analysis plan. Avoid jargon and overly complex sentences that might confuse readers. Instead, use straightforward language to describe your methods, tools, and objectives. For instance, instead of saying, “Data will be processed via multivariate statistical techniques,” specify, “We will use multiple regression analysis to examine the relationship between variables.” Simple, direct language makes your plan accessible and understandable.
Be Specific About Methods and Tools
Vague descriptions can lead to confusion or misinterpretation. Specify the exact tools and methods you’ll use for analysis. For example, mention software like SPSS for statistical analysis or NVivo for qualitative coding. Include details about the statistical tests or coding frameworks you’ll apply. This specificity demonstrates that you’ve thought through your approach thoroughly.
Align Your Plan with Your Dissertation’s Methodology Chapter
Your data analysis plan should complement your methodology chapter. Ensure the analysis methods align with the research design, data collection techniques, and objectives you’ve already established. For instance, if you conducted interviews, your plan should include qualitative methods like thematic analysis. This alignment creates a cohesive structure for your dissertation.
Seek Feedback from Supervisors and Peers
Feedback is invaluable when drafting your data analysis plan. Share your draft with your academic supervisor, peers, or mentors to identify areas that need refinement. They may offer insights into overlooked methods or suggest ways to improve clarity and precision. Incorporating constructive feedback can significantly enhance the quality of your plan.
Example of a Dissertation Data Analysis Plan
Research Topic: The Impact of Social Media Usage on Academic Performance Among College Students
Objectives:
- To explore the relationship between daily social media usage and GPA.
- To analyze differences in academic performance across varying levels of social media activity.
- To assess whether specific social media platforms have unique impacts on academic outcomes.
Methods:
- Data Collection Methods:
- A survey collected quantitative data, including hours spent on social media daily, GPA, and platform preferences.
- Demographic data such as age, gender, and major were also gathered.
- Analytical Methods:
- Descriptive statistics (mean, median, mode) to summarize social media usage trends.
- Pearson correlation to measure the strength of the relationship between social media usage and GPA.
- ANOVA to compare GPA across groups with low, moderate, and high social media usage.
Tools:
- Software: SPSS for statistical analysis.
- Other Resources: Excel for initial data cleaning and organization.
Data Preparation:
- Data cleaning involved addressing missing values using mean imputation for numerical variables and excluding incomplete responses.
- Outliers were identified using boxplots and addressed to ensure the dataset’s integrity.
Timeline:
- Week 6: Interpreting results and creating visualizations for findings.
- Week 1-2: Data cleaning and organization.
- Week 3: Running descriptive statistics and preparing datasets for advanced analysis.
- Week 4-5: Conducting correlation and ANOVA tests.
Conclusion
A well-structured data analysis plan is the backbone of any successful dissertation. It ensures that your analysis aligns with your research objectives, maintains methodological rigor, and guides you in transforming raw data into meaningful insights. By addressing potential challenges early and choosing appropriate tools and methods, you can streamline the process and enhance the quality of your research.
As you embark on crafting your data analysis plan, remember to follow the steps outlined in this guide. Define your objectives, align your methods with your research questions, and be thorough in your preparation. Incorporate the tips provided to make your plan clear, detailed, and effective.
Your data analysis plan is more than just a roadmap—it’s a vital component that bridges your research design and findings. With a strong plan in place, you’ll set yourself up for success and make the journey through your dissertation smoother and more manageable.
Need Expert Help with Your Data Analysis?
If you’re looking for professional assistance to ensure your dissertation data analysis is thorough, accurate, and aligned with your research objectives, our Dissertation Data Analysis Services are here to help. Our team of experts can guide you through every step, from data collection and preparation to advanced statistical analysis and interpretation.
Don’t let data analysis overwhelm you— Place an Order Now to get the support you need and ensure your dissertation stands out.
Frequently Asked Questions
A data analysis plan is a detailed strategy that outlines how you will process, analyze, and interpret the data you collect for your dissertation. It ensures that your analysis is systematic, methodologically sound, and aligned with your research objectives. A solid data analysis plan helps avoid inconsistencies, saves time, and improves the quality and credibility of your findings.
The choice of analysis methods depends on the type of data you collect and your research questions. Quantitative data typically requires statistical methods like regression or ANOVA, while qualitative data often involves thematic or content analysis. It’s essential to align your methods with your research design and objectives, and you can always seek guidance from your advisor or reference similar studies in your field.
Common tools for data analysis include SPSS, R, NVivo, Excel, and Python. SPSS is great for statistical analysis, while NVivo is ideal for analyzing qualitative data. Excel and R can also be used for organizing and running various analyses. The choice of tool depends on your data type and the complexity of your analysis.
Yes, our Dissertation Data Analysis Services include data preparation and cleaning. We help with organizing your data, handling missing values, identifying outliers, and ensuring your dataset is ready for analysis. Proper data preparation is crucial to avoid inaccuracies in your results.